MLOps at Scale
This MLOps system is designed to provide a full-lifecycle machine learning operations pipeline that combines experiment tracking, automated deployment, and governance under one cohesive AWS-driven architecture. It's centered around continuous integration and continuous delivery (CI/CD) principles, ensuring that models can go from idea to production safely and efficiently.
🚀 Pipeline Overview
- Experimentation & Development: Data scientists experiment using notebooks and scripts like
preprocessing.py
,train.py
, andevaluate.py
. These scripts are version-controlled and pushed to GitLab. - Source Control in GitLab: Code changes trigger GitLab CI/CD pipelines that lint, test, and validate the ML codebase. Successful pipelines result in a Docker image or script bundle that’s ready for deployment.
- Pipeline Execution in SageMaker: GitLab triggers a SageMaker Pipeline that includes stages for preprocessing, training, evaluation, and post-processing. Logs and metrics are recorded throughout.
- Model Registry: If evaluation passes, the trained model artifact and associated metadata are pushed into the SageMaker Model Registry. Versioning, approvals, and tagging are applied.
- Model Steward Approval: A designated steward receives a notification and inspects metrics, audit trails, and lineage before approving or rejecting the model version.
- Conditional Deployment:
- Approved: The model is deployed to SageMaker inference endpoints across dev, QA, and prod environments using GitLab’s environment strategy.
- Denied: The pipeline halts and the workflow is routed back to the experimentation phase for retraining or feature adjustments.
- Monitoring & Feedback: Each environment is equipped with monitoring via AWS CloudWatch and custom Lambda functions for drift detection, latency tracking, and alerting. Insights feed back into the next development cycle.
This modular architecture ensures strong governance, scalability, and observability. It empowers your ML team to iterate quickly without compromising traceability, reproducibility, or compliance.
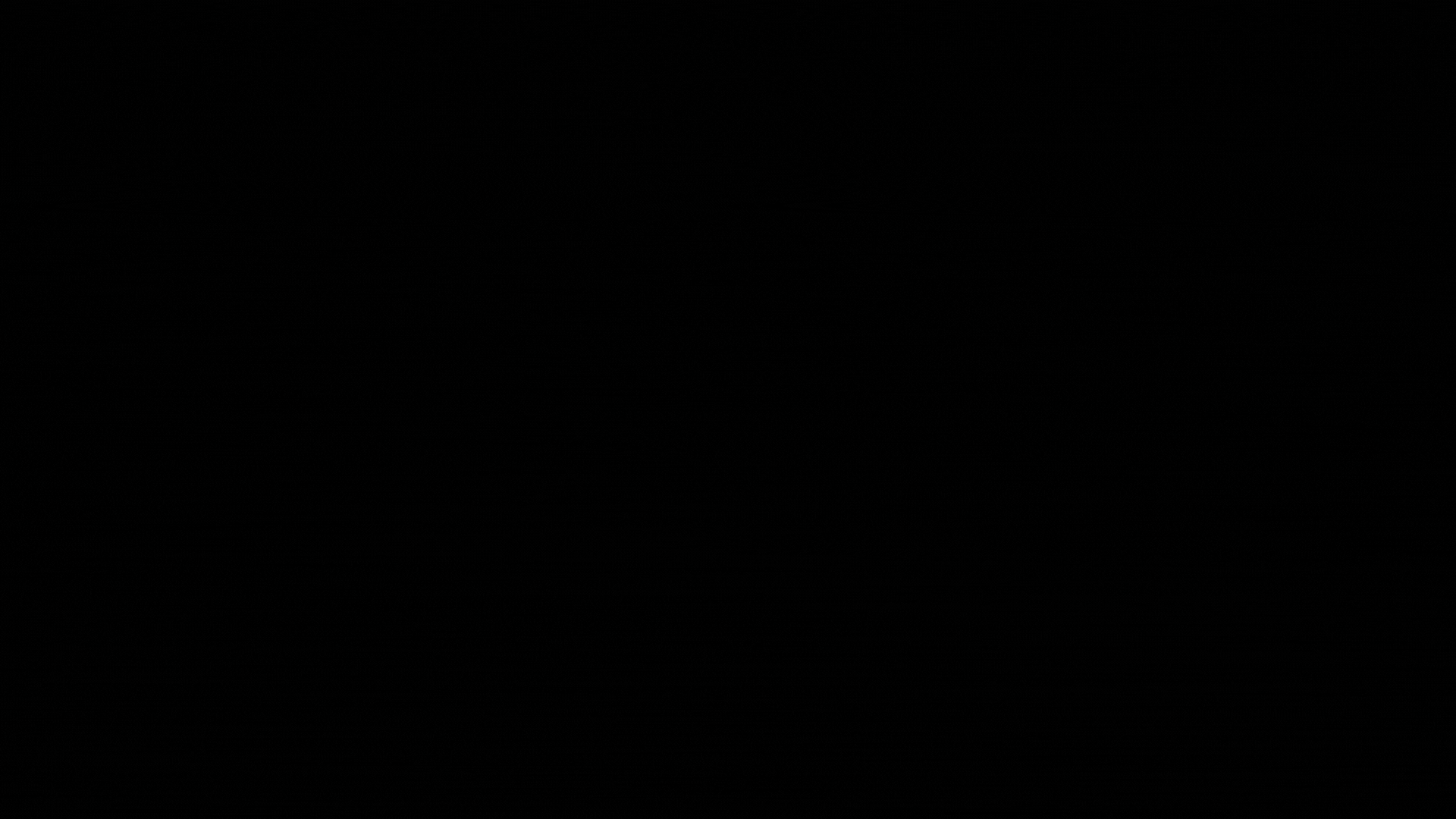
Interested in building scalable ML infrastructure in your organization?